Plant-Microbial Ecology
My work investigates how microbes are affected by their environmental conditions and in turn how this interactions between microbes and the environment impacts plant performance. I combine field collections, manipulative experiments, microbial sequencing and multivariate statistical analyses to understand these plant-microbial-environmental relationships.

Diversity and Structure of Soil Fungal Communities across Experimental Everglades Tree Islands
Everglades tree islands are an imperiled ecosystems. I collected soil samples across multiple tree islands that varied across environmental gradients and sequenced the fungal community. I found that relative water level is the greatest predictor of fungal community composition and diversity. These findings can have consequences for hydrological management decisions in the future.
Hydrology shapes microbial communities and microbiome-mediated growth of an Everglades tree island species
In this project I manipulated the hydrological conditions and microbial conditions of a tree island native strangler fig (Ficus aurea) to understand how the interactions between microbial communities and hydrology impact plant performance. I found that hydrological treatment changed microbial community composition especially of certain bacteria important for nitrogen cycling (Rhizobiaceae). These changes in microbial community also had cascading effects on plant root growth.


Foliar fungal diversity effects on host plant performance
Our understanding of the effects of foliar fungal communities on their plant hosts is limited to manipulative studies that investigate pairs or trios of fungi. In this study I manipulated synthetic communities of foliar fungi to understand how different levels of community diversity impact plant performance. There was a quadratic relationship between plant performance and number of fungal inoculants. Plant growth often responded non-additively to greater diversity communities exceeding the expected outcomes as determined by singly inoculated plants.
Not that kind of tree: Assessing the potential for decision tree–based plant identification using trait databases
Advancements in machine learning and the accessibility of "big data" have created an opportunity to improve trait based plant identification. We used decision trees inducted with 16 plant traits and assessed how well the algorithm classified species into genera from test data. 89% of species were correctly classified into their genera demonstrating the flexibility of decision trees to classify new species into genera within the tree. The proportion of information missing from the trait data was significantly related to the amount of information gained by using that trait in the decision tree. This project was done in collaboration between myself and my advisor and computer scientists.
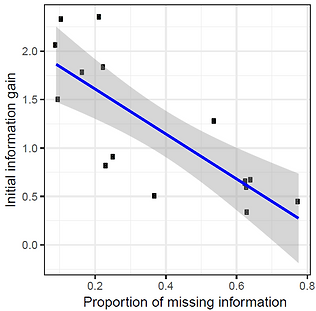